Scientists have tested several machine learning algorithms to predict the optimal tilt angle (OTA) of solar projects in 37 Indian cities, resulting in improvements of up to 90%.
An international group of researchers has used feature selection-based artificial neural networks (ANNs) with various machine learning algorithms to predict the OTA of PV systems. They conducted their experiments at 37 sites in India and depending on the ANN evaluated, the accuracy improvements ranged from 38.59% to 90.72%.
“The OTA of solar modules is one of the critical variables that determine the effective installation and operation of PV systems,” the group said. “An OTA allows the sun’s rays to be absorbed as much as possible by the material. When collecting solar energy, PV modules are affected by the angle at which light hits them from different directions. Consequently, the annual energy production of the system is directly affected by the choice of the appropriate tilt angle.”
The experiment was based on information from the NASA Power Data Access Viewer website and included the following parameters: global solar irradiance (SR), diffuse SR, extraterrestrial SR, global SR on an inclined surface, and the clarity index. They extracted data from 37 Indian cities, including New Delhi, Mumbai, Bangalore, and Kolkata.
“In machine learning and data analysis, feature selection is a crucial step that determines which variables and predictors in a dataset are most important and contribute to the predictive potential of a model,” the academic team explained. “Relevant feature selection minimizes overfitting, increases interpretability, and boosts model accuracy. See the following subsections for a list of feature selection methods.”
The team used feature selection techniques, including the Pearson correlation coefficient, to evaluate the strength of data associations and the signal-to-noise ratio to simplify the process in the presence of high noise. After applying these methods, they decided not to include extraterrestrial SR in the ANN prediction models.
“The inputs of global SR, diffuse SR and monthly average clarity index showed a stronger negative relationship with the OTA output compared to extraterrestrial radiance, which showed a negligible relationship with the OTA output,” they said. “The global SR on inclined surfaces showed a moderate positive relationship. A negative correlation means that OTA decreases as global SR, diffuse SR and monthly average clarity index increase. The extraterrestrial radiance input has a lower signal-to-noise value, which means its correlation with OTA is low.”
Next, six ANN algorithms were tested and their predicted tilt angle was compared to real OTA target values. Then, the improvement in prediction accuracy (IPA) was calculated to show how feature selection improved the mean square error (MSE) when compressed to the MSE of the calculation with all parameters.
The lowest IPA was recorded in the case of scaled conjugate gradient (SCG) showing an improvement of 38.59%. This was followed by an IPA of 53.33% in the case of Levenberg-Marquardt (LM) and 66.93% for the Polak-Ribiere conjugate gradient (PRCG). The single-stage secant (OSS) had an IPA of 86.88%, while Broyden-Fletcher-Goldfarb-Shanno (BFGS) recorded 89.53%. The Elman neural network (ELM) provided the best improvement with 90.72%.
“The models developed in this study will be used to optimize energy production, increase efficiency, and make informed decisions about solar panel tilt angles,” the scientists said. “The model will help industry participants achieve better results and promote the use of OTA by making predictions at different locations. Future research can focus on OTA predictions by using high-precision solar irradiance measurements and incorporating other factors such as dust, pollution, and aerosols. More region-specific OTA models will be developed and validated under different climatic conditions.”
They presented their results in “Novel feature selection based ANN for optimal solar panels tilt angles prediction in micro grid”, which was recently published in Case studies in thermal engineering. The study was conducted by scientists from India’s SR University, IIMT University, Government College Hamirpur, COER University, South Korea’s Gachon University and Hungary’s Eötvös Loránd University.
This content is protected by copyright and may not be copied. If you would like to cooperate with us and use some of our content, please contact us: [email protected].
Popular Content
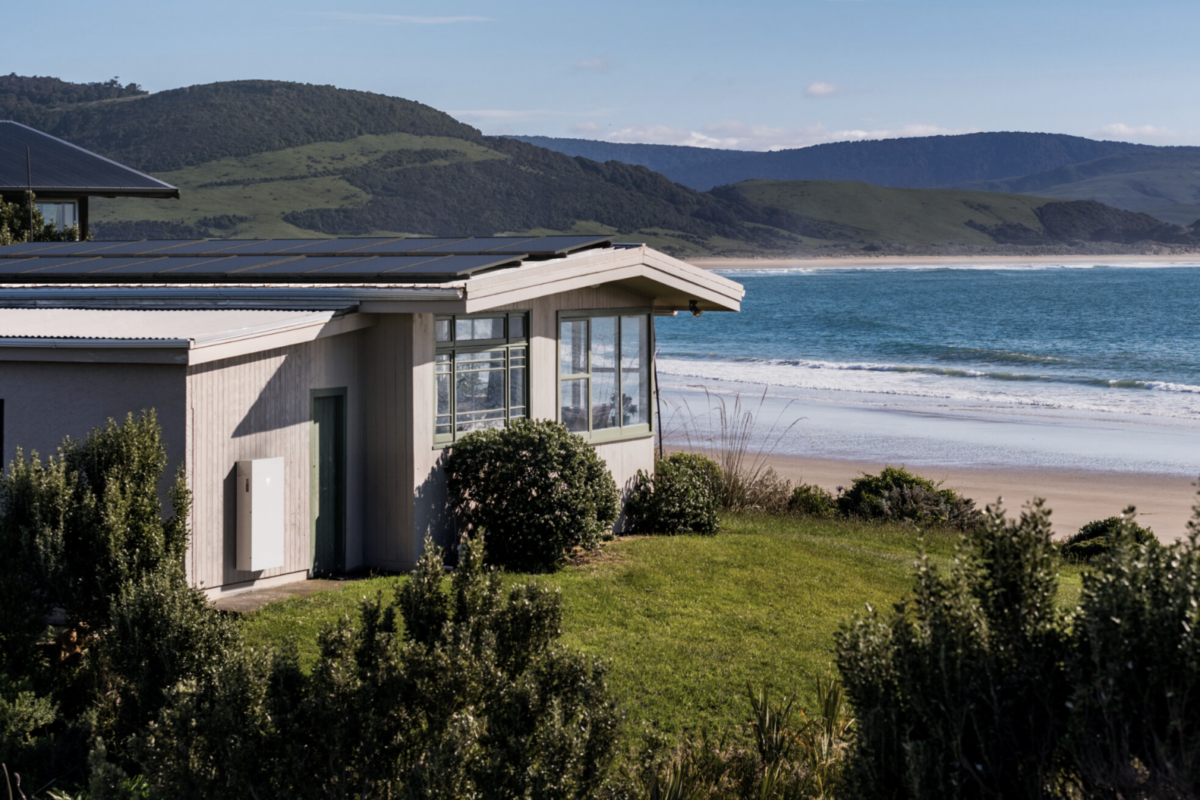